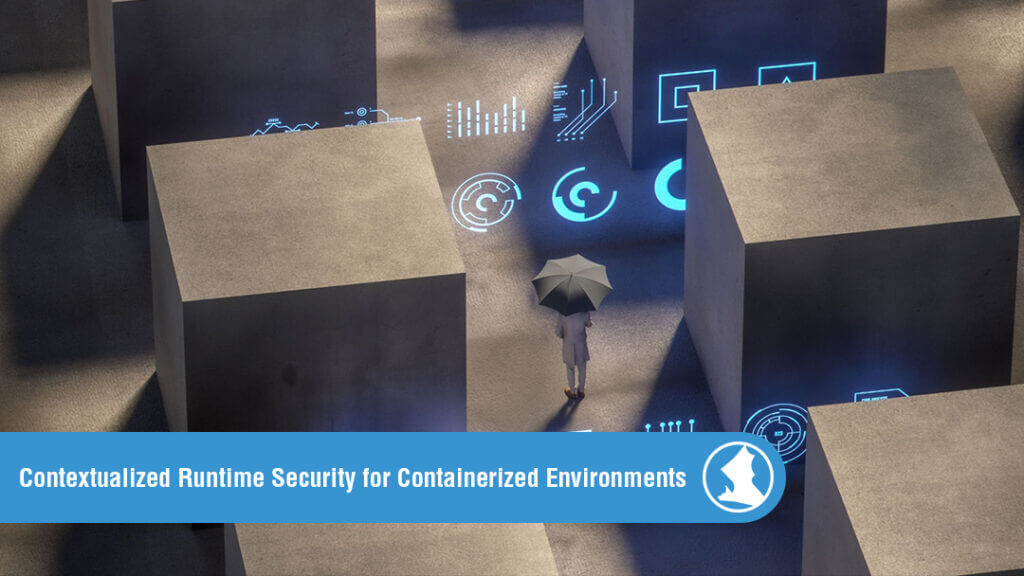
Throughout the last decade – possibly beginning with the creation of Cisco’s pxGrid framework in 2013 – security researchers have been developing tools and concepts to enhance traditional capabilities through a practice known as context-aware security. In context-aware security, analysts try to incorporate a wider field of information types, such as device locations, device types, time of the event, and IP matches from low reputation lists. The intended purpose of including this data in security investigations is to contextualize events for analysts and aid them in identifying false positives. But, does it work?
Does More Information Equal More Context?
Following nearly a decade of development in the context-aware security arena – which now includes many enterprise vendors – contemporary studies show that approximately 45% of alerts in security applications are false positives. As a result, nearly 75% of organizations report spending as much time – or even more – dealing with false positives as they do responding to real attacks, making false positives responsible for as much system downtime as genuine malicious activity.
As this alert fatigue continues to spread throughout the industry, it poses a
twofold threat to organizations. Firstly, more time spent on false positives means less time spent on real attacks, diminishing overall security effectiveness. Secondly, fatigue creates job dissatisfaction, driving down retention rates for experienced IT personnel.
With global cybercrime rates riding a three-year spike that shows no sign of relenting, it’s time for security experts to reevaluate what a contextualized view is in security investigations and ask whether the addition of more information from more sources always translates into better awareness. The fastest way to distinguish real attacks from harmless anomalies is to detect intent. Actions signal intent as one event leads to another, revealing conscious purpose.
But can analysts see intent and causal connections in the likely half dozen security feeds they’re monitoring? The trends for false positives and dwell times suggest that the answer is typically no and that even context-aware data may only add to the clutter of data overload at that moment.
Sequence-Oriented Context with Spyderbat
Spyderbat’s comprehensive runtime monitoring platform takes a radically different approach to contextualizing security events. Instead of piling on more data to be weighed in an investigation, Spyderbat captures all workload behaviors – as extracted from eBPF – in a living map called the Spyderbat Behavioral Web.
Using the Behavioral Web, operators see activities mapped to their process lineage, connecting previous processes. Spyderbat monitors every causal sequence of activities, called a Spydertrace. Spyderbat flags suspicious activities on the Behavioral Web as they occur. As flagged activities accumulate, Spyderbat raises the threat score for the entire Spydertrace .
Additionally, with the Behavioral Web Spyderbat fingerprints workload behaviors of each individual microservices. Within a microservice, there is a graspable number of processes and network connections. A key difference between a whitelist approach and Spyderbat is the Behavioral Web adds the sequence-oriented context of parent/grand-parent processes and effective user rights to the workload, enabling accurate workload behavior fingerprinting. The result is a capability that empowers developers to capture new workload behaviors between builds and arms SREs/Platform Engineers to identify new workload behaviors across environments, preventing misconfigurations or supply chain compromises.
With the ability to monitor events in a causally related sequence, Spyderbat immediately detects concerning activities while avoiding false positives. By using eBPF, Spyderbat captures activities consistently throughout the entirety of your environment, no matter how delocalized or containerized it may be.
To learn more about how to stop live attacks in your cloud-native environments and see Spydertraces in action, book a personalized demo.